Unlocking the Secrets of Human Evolution with AI Techniques
Written on
Understanding the Complex Path of Human Evolution
The journey of human evolution is intricately complex. It is not simply a linear progression from ape-like ancestors to Homo sapiens. Rather, it resembles a convoluted narrative involving various populations, each with different degrees of divergence and interaction—a real tangled web.
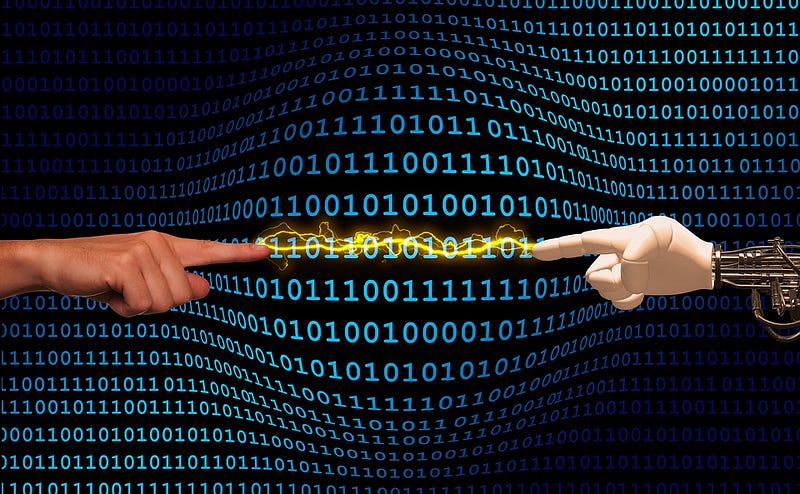
Challenging Common Misconceptions
Contrary to popular belief, evolution is not a straightforward process. It is marked by numerous twists and turns, with many alternate paths that may or may not reconnect with the original course. Notably, two prominent side paths led to the Neanderthals and Denisovans. Our ancestors were adventurous, engaging in genetic exchanges that have left traces of Neanderthal and Denisovan DNA within modern human genomes.
This transfer of genetic material between different (sub)species, which later becomes integrated into the gene pool of another species via hybridization and backcrossing, is referred to as introgression. An interesting example of this phenomenon is seen in Tibetans, who possess genetic traits inherited from Denisovans, allowing them to thrive in high-altitude environments.
Deciphering these introgressions poses significant challenges, particularly when assessing whether the exchanged genetic material serves an adaptive purpose—enhancing fitness. As highlighted in a 2019 article, the complexities of introgression can produce various genomic patterns in the recipient population, influenced by factors like migration rates and selection intensity. Given these intricate patterns, no singular method exists to identify signs of adaptive introgression.
AI's Role in Deciphering Human Evolution
Fortunately, recent advancements enable us to tackle complex data more effectively, particularly through the application of artificial intelligence. A newly published study introduces a convolutional neural network (CNN) designed to identify adaptive introgression in human evolution.
The researchers employed a method that divides the genome into 100kbp (100,000 base pairs) windows, which are then arranged into a matrix. The neural network is trained using a variety of simulated selective scenarios, utilizing these matrices as input. A notable advantage of this approach is that image resizing techniques can be applied to expedite training without sacrificing crucial data nuances.
The study tested the network under two scenarios:
- Three populations: African, European, and Neanderthal, incorporating Neanderthal gene flow into Europeans.
- A more intricate model featuring African, Melanesian, Neanderthal, and Denisovan populations, with two waves of Denisovan gene flow into Melanesians, along with Neanderthal gene flow into non-Africans.
Once testing was completed, it was time to analyze real data. The study confirmed adaptive introgression of Neanderthal genes into modern humans, validating previous candidate genes (including BNC2, 245 KCNQ2/EEF1A2 WRD88/GPATCH1, and TANC1) and identifying two new DNA regions on chromosomes 6 and 20 associated with Neanderthal introgression. Genes in these regions are linked to important biological functions such as blood cell counts.
Additionally, the researchers examined Denisovan introgression in Melanesian genomes. Similar to the previous findings, they confirmed earlier results and discovered several new regions with introgression signals. While the article details numerous regions, significant genes linked to blood disorders, tumor suppression, skin development, Alzheimer’s disease risk, and diabetes risk were highlighted.
The authors concluded:
“...we have shown that CNNs are a powerful approach to detecting adaptive introgression and can recover both known and novel selection candidates that were introduced via admixture.”
However, the efficacy of the neural network is contingent on the quality of data it processes. The timing and relative infrequency of certain introgression events can influence the classification of specific DNA segments. It is also essential to possess sequencing data from the populations from which introgression originated (in this case, Neanderthal and Denisovan DNA). The current samples of Neanderthal and Denisovan DNA represent only a fraction of the genetic diversity that existed in these extinct populations.
As research continues, it becomes increasingly evident that, despite the extinction of our sister species, their genetic legacies are intricately woven into our own genomes.
Chapter 2: Exploring AI's Impact on Evolutionary Studies
The first video, "AI, Humanity & Evolution | Q+A," delves into the intersection of artificial intelligence and our understanding of human evolution, featuring insights from experts in the field.
The second video, "Why AI will never overcome human evolution," presented by Max Bennett, offers a thought-provoking perspective on the limitations of AI in replicating the complexities of human evolutionary processes.